Our latest publication “Actuation manifold from snapshot data”, we introduce a novel a low-dimensional manifold from time-resolving flow data for a large range of operating conditions (control laws).
Published as Open Access in the Journal of Fluid Mechanics, our data-driven methodology to learn a low-dimensional manifold of controlled flows. Using isometric mapping (ISOMAP) as encoder and a combination of a neural network and a k-nearest-neighbour as decoder. The actuation manifold not only simplifies the complex interactions in the controlled flow but also reveals physically meaningful parameters, opening up exciting possibilities for flow estimation and control with minimal sensors.
The full data sets and code are freely accessible. The publication can be accessed here.
We kindly thank our collaborators from the UC3M Statistics Department and from the Harbin Institute of Technology in Shenzhen, China.
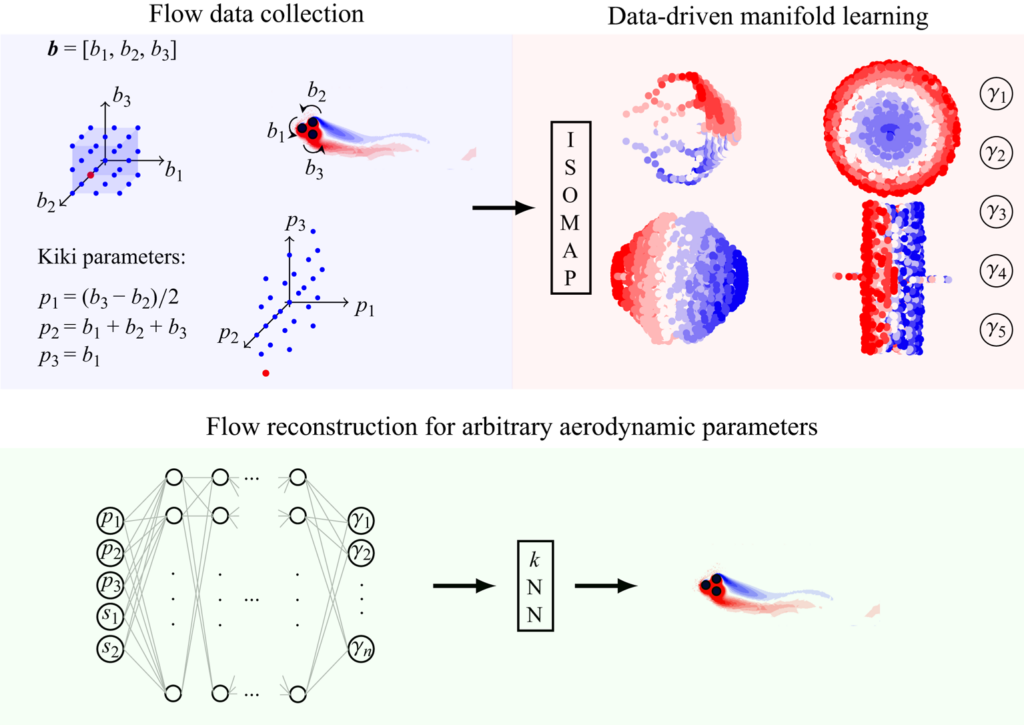