Is it possible to train super-resolution generative adversarial networks without full high-resolution samples? The answer is in our recent publication on Nature Machine Intelligence. Check it out here: https://lnkd.in/e-gpgd22
and Arxiv version here: https://lnkd.in/edQEi4Kc
with datasets (https://lnkd.in/eDgZNeMD) and codes (https://lnkd.in/eZQiK6JZ) available in open access.
Reconstructing field quantities from sparse sensors is a relevant problem in fluid flow measurements, meteorology, biological flow, air pollution control, etc. Further complexity (but also an opportunity!) arises when sensors are moving. In order to get data on a fixed grid, current techniques require withdrawing spatial resolution, or performing estimation using high-resolution dictionaries or training samples…
How can we perform this process while preserving the spatial resolution of the individual sensors?
The idea behind our Randomly Seeded GAN (RaSeedGAN) is that you can build high-resolution incomplete samples just by binning the domain with small bins, and masking out each bin where no sensors are detected. Provided the sensors randomly cover the space in variable locations from sample to sample, you can train your super-resolution GAN without any separate high-resolution complete data for training, nor introducing assumptions or models. RaSeedGAN will use the incomplete training data to establish the mapping from low-resolution data (obtained for instance from moving averages of the sensor data) to complete high-resolution fields.
This work has been carried out by Alejandro Güemes Jiménez, Carlos Sanmigul Vila and Stefano DIscetti, and developed within the Starting Grant NEXTFLOW project (https://lnkd.in/dBQKQSAk), funded by the European Research Council (ERC) (grant agreement No 949085).
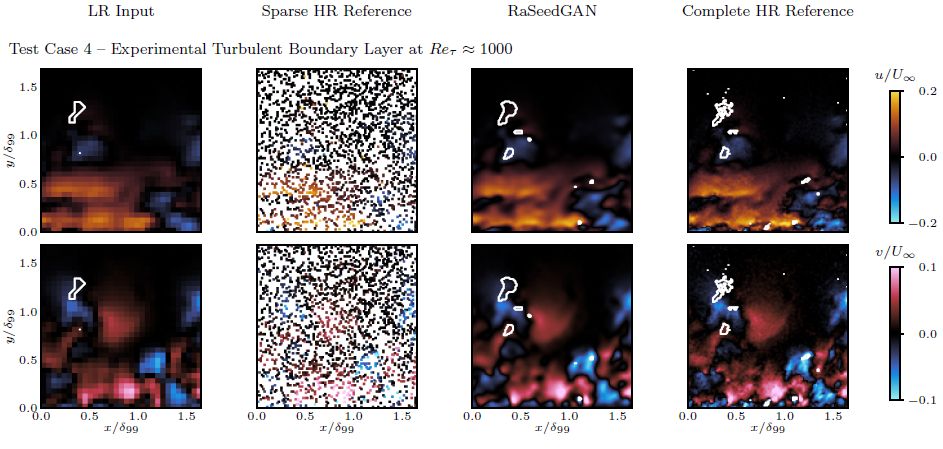